White Supremacy and Tech: Panelists Discuss Bias in Data and Algorithms
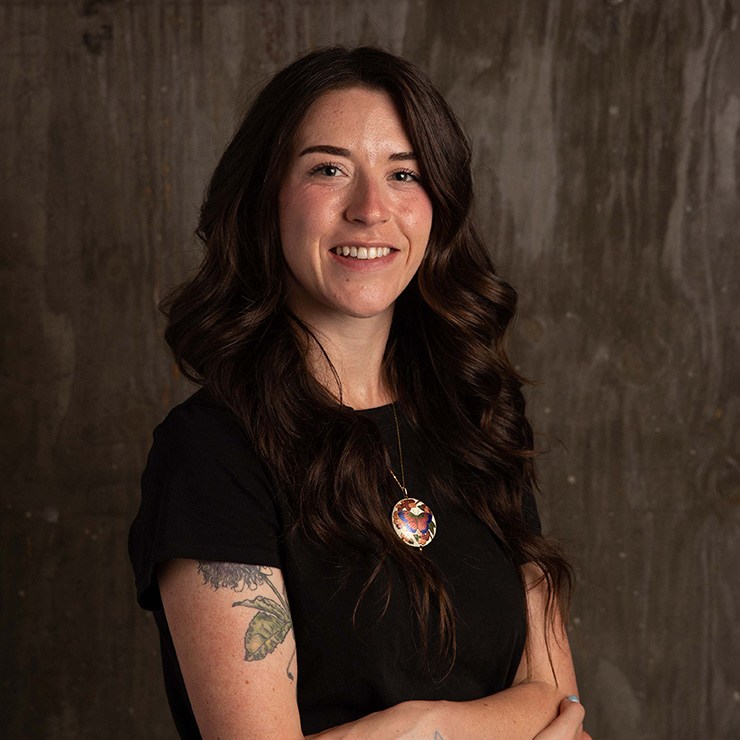
Media Contact
Danielle Bell
SENIOR PROGRAM MANAGER FOR MEDIA RELATIONS
media@greenlining.org danielle.bell@greenlining.orgThe Daily
By Thelonious Goerz
Often, data and algorithms are seen as a beacon of objectivity and fairness. But panelists in fields spanning data science, education, social justice, and policy challenged the notion with thoughtful examination last Monday.
At the event, panelists described how gender, trans, and racial biases are being perpetuated in tech, despite the popular myth that algorithms are completely objective.
The event, titled “Connecting the Dots: Racism in Algorithms and Tech,” was moderated by Haleema Bharoocha, a tech equity policy fellow at the Greenlining Institute from Oakland, California. Bharoocha co-hosted the event with the Greenlining Institute, the Critical Platform Studies Group, and UW’s Information School.
Panelists included Nikkita Oliver, a case manager and former Seattle mayoral candidate; Shankar Narayan, director of the ACLU of Washington Technology and Liberty project; Anna Lauren Hoffmann, professor at the UW Information School; and Pedro Perez, co-founder of Geeking Out Kids of Color (GOKiC).
“Technology, often framed as apolitical, reaches into the lives of anyone whose lives are mediated by networks or data analysis,“ Bharoocha said. “Algorithmic bias goes beyond big data concerns: facial recognition technology … can replicate racial bias by reproducing historical injustices from the data sets they are built from.”
While it may seem that data doesn’t “lie,” Hoffmann commented on the nature of asking the right questions when collecting and using data. For Hoffman, bias in data comes from the way we collect our data sets, which are often exclusionary, and make people “data invisible.”
This was most recently apparent in Amazon’s hiring practices. Using artificial intelligence, Amazon created an algorithm to compare and review the resumes of prospective employees against the resumes of their current employees.
Because the majority of Amazon’s employees are white and male, the data set produced a pool of prospective employees that reflected that demographic. According to an article in Business Insider, the algorithm discriminated against women, going so far as to exclude any candidates that went to certain women-only colleges.
The same type of discrimination and bias can be seen in more extreme situations as well. Notably, panelists discussed the predictive policing tactics that the Seattle Police Department (SPD) had used until recently. According to Oliver, SPD uses the crime data to determine the “hot spots” for crime, and as a result, determine where to increase police presence.
Oliver also spoke about a group of community organizers in Seattle that used the same SPD data to determine where to perform outreach and community engagement, which led to a reduction in crime. In this way, Oliver characterized data as a tool that could be used to either criminalize a population or help a population through outreach.
In terms of surveillance, technology does not stop with predictive policing; it also extends to facial recognition. Narayan argued that the way tech is marketed as being neutral is actually misleading, as it can actually have detrimental impacts on communities of color.
Narayan called facial recognition a “supercharging of racism,” as it determines propensities for violence, anger, and whether someone is a terrorist. The problem with these algorithms, according to Narayan, is that these technologies are not able to be evaluated by third parties before use. Some of this is due to the nature of black-box and proprietary technologies, which are often kept secret so as not to expose novel technology to competitors.
Narayan pointed to the need for regulation and policy surrounding these systems, especially when they claim to be able to predict certain traits.
While this characterization can seem grim, Perez offered some positivity about the emerging future of technology and algorithms.
Perez is the co-founder of GOKiC, an organization that provides children of color with more access to computer science and tech. Through after-school resources and workshops, Perez teaches young children about coding in an inclusive and socially conscious environment. According to Perez, GOKiC uses examples to teach computer science that engage kids culturally, material which he finds to be more resonant.
Perez further explained that a lot of youth have limited access to technology. Many of the children that GOKiC serves don’t have a computer at home, which impacts their school performance, according to Perez. These barriers further disadvantage children of color and contribute to maintaining inequality.
At the panel’s conclusion, Hoffmann noted that data and algorithms should be used to challenge white supremacy and the status quo. Rather than asking how we can modify the algorithm to be fair, Hoffmann urges tech workers to also look at the system that the algorithm represents, to look beyond what is already on the surface.