Race-Aware Algorithms: Paving the Way for a More Equitable Future
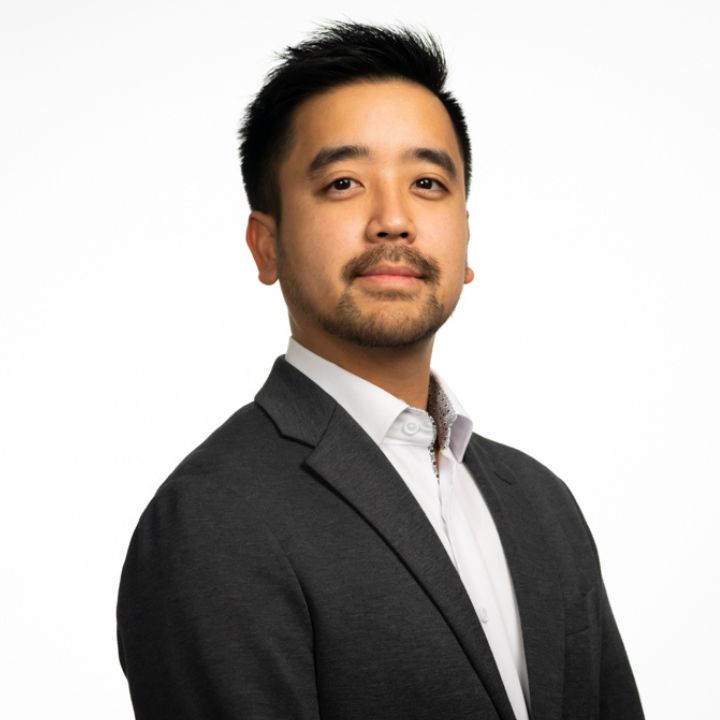
In a tech-era dominated by algorithms and AI, automated decision systems (ADS) have become an integral part of our lives. Algorithms have the potential to streamline processes, reduce human biases, and improve efficiency – but they don’t always work properly. And, when these systems are entrusted with making decisions that significantly impact our lives, the stakes become incredibly high. This is especially true for communities of color that face systemic discrimination. History has shown that these systemic biases can seep into decision-making processes. Automated systems, if not carefully designed and monitored, can perpetuate these biases. For instance, a system that lacks adequate representation of diverse and underrepresented groups in its training data might discriminate against certain groups, such as women and people of color – perpetuating systemic discrimination.
The Dilemma: Privacy vs. Accuracy
One of the major dilemmas with the use of ADS is the depth of personal information these systems require to make accurate decisions. While providing race and ethnicity data might lead to systems that are better equipped to identify and mitigate bias, it also introduces risks about privacy and potential misuse. This is further complicated by a history of businesses using race data to make discriminatory decisions, such as banks redlining entire neighborhoods for disinvestment based on racial classifications. Civil rights-era legislation then barred institutions from discriminating based on race, ushering in an era of ignoring race altogether. The Supreme Court’s recent affirmative action ruling, for example, helped reinforce this race-unaware status quo in education despite the effectiveness of race-aware admissions policies in redressing historical discrimination. However, this decision does not close the door to using demographic data in ADS to make decisions, particularly when it is used to test systems for bias and to improve fairness and accuracy.
In 2021, The Greenlining Institute published Algorithmic Bias Explained: How Automated Decision-Making Becomes Automated Discrimination, where we urge policy- and decision- makers to explore the use of race-conscious or race-aware algorithms and AI systems as opposed to the models often used today that do not consider sensitive characteristics like race and gender.
These recommendations are based on research that shows allowing algorithms to access data on race and other characteristics can help make more accurate decisions and prevent discrimination because including this data can allow developers to determine if there is a lack of representation in their datasets and to de-bias their models. We also found that algorithms can discriminate by using other variables as proxies for race, for example using your internet history, last name, or your zip code – so a race-unaware algorithm doesn’t always work well to prevent bias and discrimination.
Informed Transparency and Accountability
In Race Aware Algorithms: Principles and Policies for a More Equitable Future, a report authored by Alice Lee in consultation with The Greenlining Institute, we interviewed eight AI fairness experts from business and civil society on the use of race data in ADS. The report makes the case that race data can and should be used in different contexts to improve equity and fairness in automated decisions.
While the inclination might be to avoid the inclusion of race and ethnicity data to protect privacy and against intentional discrimination, it is vital to recognize that without such information, the very biases we seek to eliminate can be perpetuated through ADS. Unlike humans, algorithms are not inherently biased. Instead, bias is introduced to these systems by flawed assumptions in the algorithmic model, systemic inequities reflected in the dataset, or underrepresentation in training data that can work to perpetuate existing disparities. Race-unaware algorithms can still learn from discrimination embedded in a dataset by using proxies for race which include where you live, employment, your credit card history, last name or education data. On the other hand, access to race data can allow developers to test their models to identify unfair biases in the performance of their systems across demographic groups and to address those disparities, generating greater fairness and accuracy. Moreover, disparate impact testing using race data can help hold businesses and governments accountable legally and financially for biased decision making.
The experts we spoke to for the Race Aware Algorithms report supported the collection and usage of race data in ADS as a way to improve accuracy and reduce bias. This does not mean sacrificing privacy altogether. Instead, the solution lies in establishing strict guardrails and robust protections around the collection and usage of race data. Stringent data collection practices, transparent algorithms, thorough audits, and continuous monitoring can help ensure that the data provided is used solely for addressing biases and creating fairer systems.
Recommendations and the Way Forward:
The debate surrounding the inclusion of race and ethnicity data in ADS is highly nuanced, with real-world consequences for individuals and communities. A one-size-fits-all approach won’t suffice, and context matters immensely. To tackle biases head-on and create systems that truly level the playing field, careful consideration of race data is necessary.
Our recommendations from Race Aware Algorithms: Principles and Policies for a More Equitable Future on how both the private sector and government should approach this issue include:
Private Sector
Utilize race data to advance racial equity: Demographic data is crucial for racial bias testing. The voluntary collection of demographic data, with the appropriate safeguards, would help auditors and fairness researchers test for disparate impacts to communities of color and enable designers to better account for biased variables in an algorithm’s design.
Contextualize fairness: Businesses should contextualize their approach to fairness by disclosing how they test and measure fairness, the limitations of their systems, and how they account for sociological and demographic differences in their training data.
Track and report the impact of race on an algorithm: As developers build and test different ADS, they should systematically track and report the impact of race on the algorithm’s outputs. This can allow decision makers to make informed decisions on which model to deploy to minimize the risk of biased and inaccurate decisions.
Optimize for racial equity: Institutions using ADS should be expected to go through a careful model selection process, integrating a fairness and/or equity definition into the final model selection criteria.
Public Sector:
Apply a ‘rights-then-risk’ based framework: Advancing technology policy should always consider human rights first. Only once our rights are protected can policymakers apply a risk-based framework for regulation. In areas protected by Civil Rights laws – such as employment and credit – higher risk ADS should be assigned “special provisions” depending on the algorithm’s potential impact on people’s lives. These provisions can range from greater audit and risk management requirements or an outright ban.
Set standards for data collection and privacy safeguards: The Greenlining Institute recommends setting a long-term goal of collecting race data through voluntary self-reporting, generating higher response rate through the proliferation of data trusts or data cooperatives, and data governance approaches that strengthen the power people have on deciding how their data is provided, used, and stored. In order to reach this goal, the government should set clear, context-specific standards for race data collection and play a heavy role in ensuring the proper privacy safeguards are in place. The government should also detail the appropriate entity that is best positioned to collect, store, and use this data.
Require auditing in civil rights protected contexts: Audit requirements would put the responsibility on private firms to test for social biases. Consumer protection agencies, in collaboration with stakeholders, should take the lead in creating industry-specific policy guidelines for thorough and effective audits. Algorithms should be tested against a standard set of guidelines for the relevant sector, as well as against a specified fairness metric pre-defined in the firm’s transparency documentation. The audit should also test for multiple models and ensure the least discriminatory model(s) are selected.
Assign and equip government institutions to regulate ADS with ongoing multi stakeholder consultation: As the technical capacity of government staff and assigning responsible bodies grow, we recommend they use their authority to lead data collection and auditing. This would require expanding the scope of institutions like the FTC who have been leading on AI regulation, or creating a new federal AI agency working in collaboration with existing agencies like the Department of Housing and Urban Development, the Equal Employment Opportunity Commission and the Consumer Financial Protection Bureau. It is also critical for the assigned governing body to develop a consultative process that continually engages with multi stakeholder representatives of civil society as experts in the societal implications of technology, centering most impacted communities.
Update anti-discrimination laws to reflect algorithmic decision-making: Anti-discrimination law must be more broadly adapted to capture the many ways discrimination can occur through algorithms and to drive greater usage of race data to protect against discrimination and bias. For example, existing regulations like the Equal Credit Opportunity Act’s Regulation B should be revised to encourage increased collection of demographic data for bias testing in non-mortgage lending.
As lawmakers and policy leaders grapple with this swiftly evolving technology a multifaceted approach is required, involving the collaboration of technologists, policymakers, ethicists, and the communities affected. The road to equitable ADS is paved with the integration of race data, but it’s essential to couple this with rigorous oversight, transparency, and a commitment to protecting individual privacy. Only then can we harness the full potential of technology to create a more just and inclusive society.
This blog is based on a report which was authored by Alice Lee, a graduate student policy consultant as part of a master’s thesis at the Goldman School of Public Policy.
If you are interested in learning more about this topic, you can read the report here.
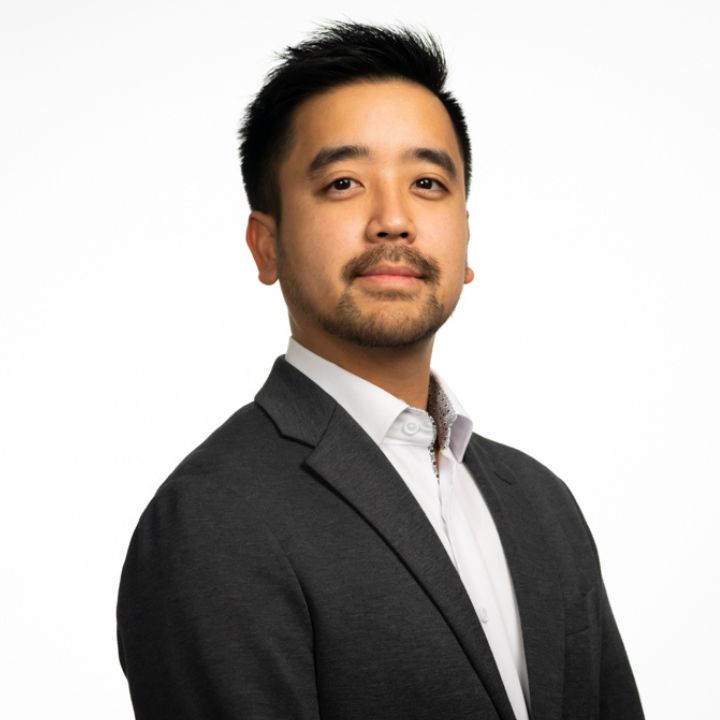